The Impact of Automation in Data Science: Opportunities and Challenges
The Impact of Automation in Data Science: Opportunities and Challenges
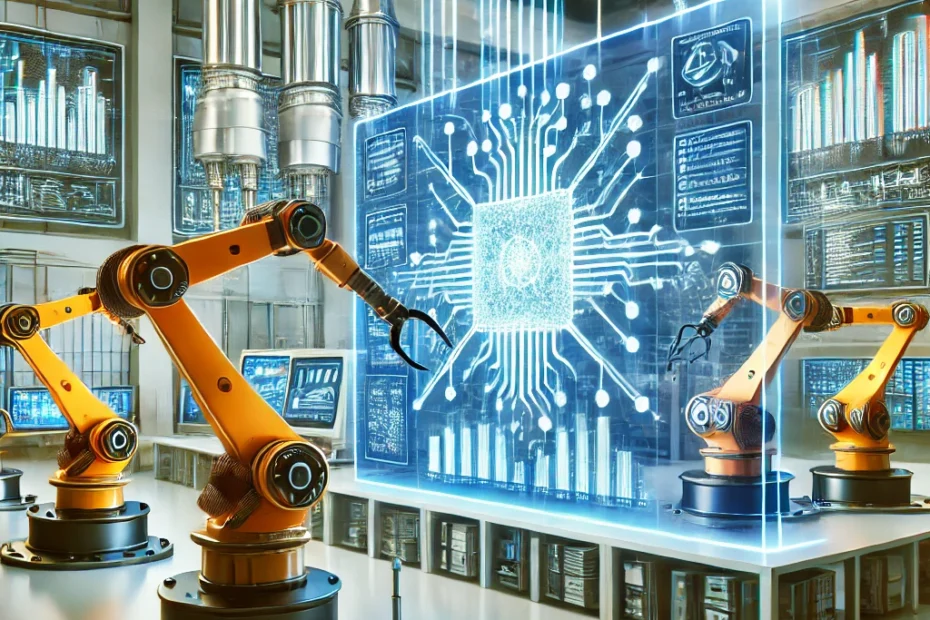
In the information age, where data has become the “black gold” of the 21st century, Data Science has emerged as a key discipline for companies aiming to stay competitive. However, as data volumes explode, a new trend is emerging: automation. It promises to make processes more efficient, reduce human errors, and accelerate data analysis. But this revolution raises important questions. What are the real impacts of automation in Data Science? What tools enable this transformation, and how can professionals adapt?
Automation: A New Paradigm in Data Science
For a long time, Data Science relied on the meticulous work of data scientists, experts capable of transforming raw data into valuable insights. Their daily tasks involved collecting, cleaning, analyzing, and interpreting data—a complex and time-consuming process. The arrival of automation is disrupting this model.
Automation in Data Science involves using software and algorithms to perform tasks that were previously done manually. From data collection to training machine learning models, and even predictive analysis, almost every step can now be partially or fully automated. This evolution not only saves time but also improves the accuracy of analyses.
Opportunities Created by Automation
The benefits of automation in Data Science are undeniable. First, it allows for the processing of much larger volumes of data than before. Where a human data scientist might be overwhelmed by millions of data rows, a machine can analyze them in minutes, or even in real-time.
Secondly, automation enhances the reproducibility of analyses. When processes are automated, they can be repeated identically, reducing errors and ensuring consistent results. This is particularly important in sectors like finance or healthcare, where even the smallest error can have serious consequences.
Finally, automation opens up Data Science to a wider range of professionals. “No-code” or “low-code” tools now allow individuals without deep programming skills to create predictive models, analyze data, and generate reports. This democratization of Data Science could revolutionize many sectors by enabling more diverse teams to contribute to the transformation of data into actionable knowledge. Now, a marketing manager or financial analyst, without being a programming expert, can use automated tools to create predictive models, segment markets, or identify hidden trends in data.
Challenges Posed by Automation
However, this increased automation is not without significant challenges. The first, and perhaps most obvious, is the fear that machines will replace humans. While automation allows certain tasks to be performed faster and with fewer errors, it could also render some skills obsolete. Data scientists must therefore evolve, focusing on more strategic tasks that require human expertise, such as interpreting results, making decisions based on complex analyses, or communicating insights to non-experts.
Another challenge is related to data quality. Although automation can process massive volumes of data, the quality of that data remains crucial. Biased or poorly cleaned data can lead to erroneous results, even with the most sophisticated tools. This means that data scientists must always exercise constant vigilance in the data preparation phase, even if this step is partially automated.
Moreover, automation can sometimes lead to an over-reliance on tools, at the expense of a deep understanding of the underlying algorithms. Automated tools can simplify the modeling process, but they also risk creating “black boxes” where users apply models without truly understanding how they work. This opacity can be dangerous, especially in contexts where decision transparency is essential, such as finance or justice.
Tools for Automation in Data Science
To take advantage of automation, it is crucial to be familiar with the tools available on the market. Among the most popular are platforms like DataRobot, H2O.ai, and Azure Machine Learning. These tools allow for the automated creation, testing, and deployment of machine learning models, reducing the time needed to go from idea to implementation.
Other tools, such as Alteryx or RapidMiner, offer intuitive interfaces that allow non-technical users to manipulate data, create processing pipelines, and perform complex analyses without writing a single line of code. These platforms bring the power of Data Science to everyone but also require an understanding of best practices to avoid common pitfalls.
Skills Needed to Adapt
With automation, the skills needed to excel in Data Science are also evolving. While mastering programming languages like Python or R remains an asset, it is equally important to develop skills in result interpretation, Data Science project management, and insight communication.
Moreover, understanding the fundamental concepts of machine learning and artificial intelligence remains crucial. Even with automated tools, a good data scientist must be able to assess the relevance of a model, detect potential biases, and understand the limitations of the algorithms used.
Finally, the ability to continuously learn has become a key skill. The field of Data Science is rapidly evolving, and professionals must stay up to date with the latest technologies, methodologies, and automation tools to maintain their competitive edge.
The Future of Automated Data Science
In conclusion, automation in Data Science is both an opportunity and a challenge. It promises to make processes more efficient, broaden access to Data Science, and enable faster and more accurate analyses. However, it also requires professionals to adapt, develop new skills, and remain vigilant against the potential pitfalls of automation.
The key to success lies in balancing the use of automated tools to enhance efficiency while maintaining human expertise to ensure the relevance and quality of analyses. The data scientists of tomorrow will not just be technicians but strategists capable of navigating an increasingly automated landscape while keeping an eye on the details that matter.
The future of Data Science is undeniably automated, but it is also a future where human expertise will remain at the heart of innovation. Those who can combine the two will be the leaders of this new era of Data Science.
Do you want to take your skills to the next level today?
Discover the future of data training. With Datakoo, every lesson is an opportunity, every module a step towards excellence. You have the potential; we have the tools. Start your transformation today!